Why did Buffer's social referral traffic vanish?
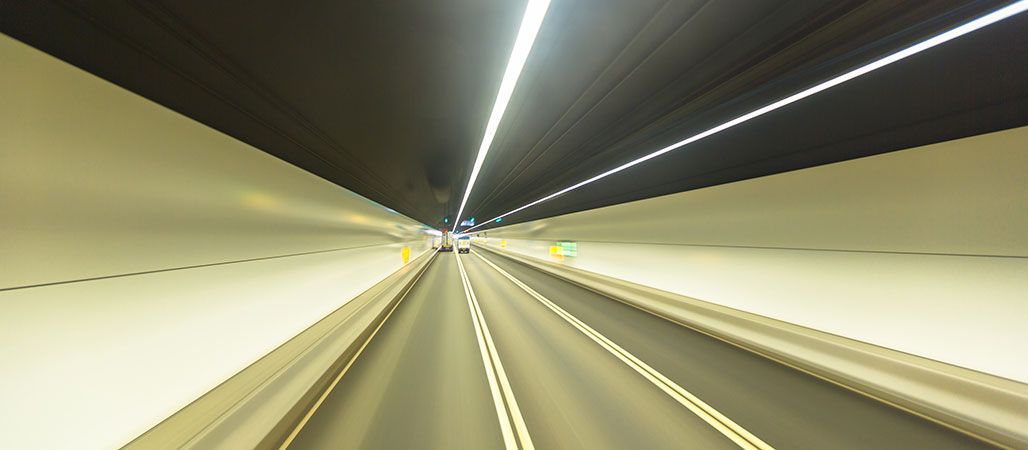
Buffer say that they lost nearly half of their social referral traffic in the last year. I’m going to do one of those annoying things and not answer the question I pose in the title. I don’t have access to Buffer’s data so I can’t give you any real insights.
What I will do is give you some ideas about how to dig deeper into these kinds of “oh my!” moments that you find in your analytics data. Sometimes it’s too easy to take figures at face value, panic and draw the wrong conclusions.
I love how open Kevan and Buffer are in their blog post. Buffer are often open, which is part of the appeal of their blog posts for me. In this post Kevan shares some pretty scarey figures – their social referral traffic has fallen through the floor!
Oh my!
The number of sessions attributed to social platforms is down 45% year on year according to the article. 118,287 sessions compared to 215,097.
Buffer also share a breakdown by individual platform. Twitter down 43%. Facebook down 53%. LinkedIn down 45%. Google+ (perhaps least surprisingly given the platform’s toning down by Google) down 72%.
The downturn has left Buffer puzzled. Kevan presents a few speculative ideas in the blog post. One of them is the idea that social media itself has changed and is still changing.
We’ll put Buffer’s suggestions to one side and go through some steps you could should take if you were faced by Buffer’s figures and wanted to get a better understanding of what was happening and feel more confident about your conclusions.
Choosing time periods
Choosing time periods to compare is critical. If you choose the wrong time period, then you skew your data and end up now comparing like with like. In their example Buffer compares a 30 day period with the same dates in the previous year.
One of the first rules of choosing time periods is making sure you match weekdays. I can see from the graph (and without checking in the calendar!) that the days of the week are misaligned by one day – So Tuesday this year is compared with Monday last year.
There is a weekly pattern with a dip on Saturdays and Sundays (more pronounced last year than this year). Shifting the dates used for the comparison by one day would make the periods align. It would then be easier to compare. It would also show that rather than 29 days out of 30 outperformed this year, all 30 days did.
This at the moment is a specific comparison between two 30 day periods. I’d also want to check that this trend was observable at other time periods. We can’t be sure at the moment if October 2014 was a blip or if October 2015 was a blip. In fact, they could both be blips!
Out of the ordinary happenings
What do we know about other happenings during the two time periods? Were there any extraordinary happenings? Examples are product launches, trade events, newsletters, links from media sites, tweets by influencers. These can all lead to spikes.
Sometimes you might want to avoid comparing dates which include unusual happenings. You might want to filter them out separately. They have a story to tell, but perhaps it’s a different one and worthy of its own analysis.
When comparing a period with another containing a “one-off” happening, you’ll see lots of green coloured improvement stats in Google Analytics. (or lots of red worsening stats if your spike was in the older time period.) It may look pretty but it doesn’t tell you much more than you had a one-off event that boosted your figures.
Comparing like with like
In this case, we’re looking at a blog. Something that is important to know is what content was published. Say that no new articles were published this year, but 8 were published this time last year. Perhaps it’s not at all strange that social referrals are down?
They published 23 blog posts during the time period looked at this year. 20 during the same period last year. That’s similar enough. I’d be happy comparing those periods.
A tip here is to add annotations to Google Analytics to keep track of important happenings. In the case of a blog, like this one, I’d be making a note of each time a new post is published.
The article only shares figures about the number of social sessions. But what about the overall number of sessions? Have they gone down in total? If not has one source gone up as the social sources have declined? If this is the case, perhaps the audience has changed habits?
Or perhaps there has been a technology change that visualises itself as shift in source? An example of this would be if a site that normally sent you a lot of referrals moved from http to https only. Your site remains on http.
These secure to non-secure referrals may appear as direct in your reports – I’ll come back to the issue of direct traffic further down.
Further reading: How to use Google Analytics annotations
Broken analytics setup
Something I would have checked before anything else is if the analytics setup is broken. Often if something can’t be explained, it’s due to a broken setup – and I’ve seen lots of broken setups in my time.
It’s unusual to find a site where there isn’t something foobared.
Sometimes it’s not obvious that something is broken. Multiple instances of the tracking code is one such thing that produces weird effects but that isn’t always immediately obvious. Especially if the problem is only on a sub-set of pages.
Further reading: 15 ways your Google Analytics might be broken
Campaign tagging
Buffer make extensive use of custom campaign parameters in their URLs. This helps them feed more information into GA about the way links are used and travel. These parameters can’t break your setup but they can mess around with your data.
These parameters populate the source medium and campaign for a visit in Google Analytics. They override any existing source and medium that is stored for that visitor.
This means that if the medium is actually social and the source is twitter.com you could override that with any source and medium of your choosing. A medium of time-travel and a source of Tardis if you fancy.
Further reading: Social Media Campaign Tracking With UTM Parameters in Google Analytics
In Buffer’s case they have added utm campaign parameters to the top navigation links on their blog. It’s unusual to add these to links in the navigation of a site. Presumably they have been added as a way of tracking movement between sub-domains.
The “social” menu item is the home page of the blog. The link includes “blog” as the source and “social” as the medium.
If you arrive from Twitter and then click on that link in the menu, your source and medium will be updated. It will no longer look like you came from Twitter.
They will also lose the “social source referral” flag in the analytics data meaning that the session will not be included in the social report under acquision in Google Analytics.
I’m going to guess (as I don’t have access to the data!) that it’s unlikely that all the “lost sessions” could be because of this. but some will have visited the blog then at some point clicked on the link in the menu.
Direct traffic & “Dark social”
There are a whole load of situations in which visits end up being counted as “direct”. If a source is already stored in the visitor’s cookies then the direct visit won’t make a difference (at first). GA uses the last non-direct source for attribution.
Further reading: Direct visits and Google Analytics Attribution Precedence
If visitors are regularly coming to the site via a “direct” method, then over time – 6 months by default – the campaign cookie will start expiring. These visits will start surfacing as direct and there will be a drop in other sources.
If Buffer has altered the default setting from 6 months to something lower, then it accelerates the effect. It will also accelerate if users have a tendency to delete cookies (or change device).
Direct visits can take many forms. People rarely type long URLs into browsers by hand. They can be visits from places such as bookmarks, browser history, email, documents, apps, SMS, chat clients, https to http traffic.
Perhaps visitors have discovered the blog via social media (and did that in great numbers during 2014). Then moved to following the blog in other (direct) ways. Maybe Dark social got a lot darker for Buffer?
Dark social refers to the phenomenon where a link is shared and then consumed in ways which strip it of referral information, making it difficult to track and analyse where the link and the visit came from.
Buffer are good at adding campaign tags to their links (not surprising given that it’s a feature of their product!) so perhaps this is not so likely.
Segmentation
One of the first laws of web analytics is All data in aggregate is crap.
Shame on me for bringing you this far into the article before mentioning segmentation. Buffer don’t say, but it feels like no segmentation is being applied to the data when looking at the drop in social referrals.
Okay, so segmentation isn’t going to magically make 100,000 sessions reappear as social referrals. Slicing the data though might reveal groups of visitors or types of behaviour that hasn’t suffered from the same kind of downward trend.
One of the most obvious ways of segmenting the data is to look at mobile and desktop visits separately. Perhaps mobile visitors are still finding their way to the blog via social channels as often as ever?
Further reading: Segment analysis examples and How to use Google Analytics Advanced Segments
Miss Scarlet in the ballroom with the dagger
It wasn’t my intention to try to “solve” Buffer’s mystery. I wanted to give you some inspiration and a taste of the due diligence needed when looking at web analytics.
Google Analytics and similar tools are designed to make reports and data accessible to the layman. They appear to surface “facts” easily and present them in a straight-forward way.
The truth is, the data beneath the surface is really complex. It is affected by many factors both obvious and obscure. Take your time to investigate, question and understand. Don’t panic.
James is in the process of writing a book – UX Analytics. A book for UX practitioners who want to learn how to make informed decisions about the UX of websites, products or services based on UX research using web analytics data. Find out more here and sign up for updates.
Why did Buffer's social referral traffic vanish? https://t.co/6ksJ9UStSB
— James Royal-Lawson (@beantin) October 30, 2015
Featured image by IQRemix
Cluedo photo by Tim Ellis
Sith photo by East Mountain